SeqDAIS
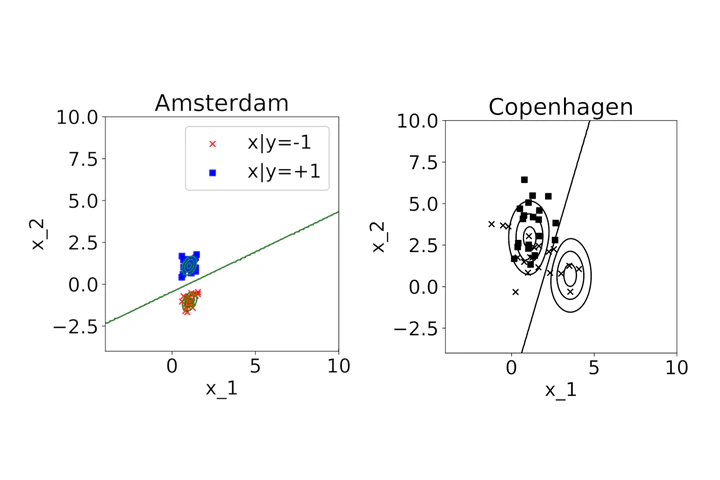
The capacity of supervised learning systems to generalize to new examples is inherently limited by the collected data. If it is not an accurate reflection of the population, then the system will not perform well. In particular, if the collected data is biased, which means that one observes certain examples more often than normal, then the system can be misled into thinking that certain outcomes are also more likely to occur. Although the system might make accurate diagnoses for new patients arriving to that particular hospital, it will make inaccurate diagnoses for patients arriving to 1a different hospital. The differences between patient populations might be due to regional differences such as exercise culture, but the fact that one observes for instance older patients more than normal means that data collected from that hospital is biased with respect to the total human population.
Handling biased samples from populations is a problem that has long been studied in statistics and econometrics. Although a number of techniques have been proposed to improve supervised learning systems trained on biased data, things have changed with the tremendous increase in computational power in the last 20 years. The field has advanced to the point where we ask the question whether it is possible to generalize to particular target populations as well. Can we adapt a supervised learning system trained on adult human heart disease patients to make accurate decisions for infant heart disease patients?
Work from the last 10 years has looked at incorporating unlabeled data from these target populations. With this additional information, systems can recognize changes in data properties, find correspondences between populations and adapt their decisions accordingly. Successful adaptation is defined as an improvement over the performance of the original system. Nonetheless, the analysis of this problem is not complete, and it is not clear which conditions have to be fulfilled in order for the system to perform well. It seems that in cases where it is difficult to describe how two populations relate to each other, adaptive systems suffer from high variability. They are highly uncertain about their decisions and often wrong. However, it seems that the more similar the populations are, the likelier it is that the system adapts well. It would, for example, be easier for the system to adapt to predict heart disease in adolescents based on data from adults, then it would for the system to adapt to infants. However, here might lie a potentially crucial insight: can we design a system that first adapts to the closest population and only then adapts to the final target population? In other words, a system that sequentially adapts?
This position is supported by a Niels Stensen Fellowship grant, offered by the Niels Stensen Stichting.